Artificial intelligence has taken the world by storm and the systematic review world has been no exception. At Covidence, we have recently introduced active learning, a machine learning model developed by EPPI-Centre, to the platform and it’s about to change the way you complete systematic reviews for the better. Now, when you start title and abstract screening in Covidence, our active learning tool can streamline the process and help you get to full-text screening sooner.
Selecting the most relevant first.
When you choose to sort studies by “Most relevant” in the Title and Abstract section of your review, Covidence displays the studies that are most likely to be included first.
How does Covidence machine learning work?
Machine learning analyses patterns in past screening behaviour in a review, and uses them to predict the relevancy of studies that have not yet been voted on and to sort them in that order. At least 25 studies must be marked as included or excluded, with at least two of each. The more studies you screen, the stronger the system’s prediction will be.
How does machine learning help me?
By leveraging machine learning algorithms, teams can quickly identify relevant studies and move them through to full-text review much faster than traditional methods. The use of AI in the screening process offers numerous benefits for teams conducting research. It saves time and resources, and improves accuracy as more data is collected, allowing teams to focus their efforts on more important tasks. By leveraging the power of machine learning, teams can work smarter, not harder, and get the most out of their research.
The sooner relevant articles are identified, the sooner their data can be used. Covidence’s flexible workflow means that team members can work across the title and abstract, full-text, and data extraction sections simultaneously to get the project finished in less time.
Do I need to screen all the studies?
The predicted relevancy scores of the articles will become lower as screening progresses. Covidence does not recommend any criteria or rules for stopping screening before all studies have been voted on. And Covidence does not remove any studies from the screening list on the basis of their predicted relevancy score.
The time saving offered by Covidence’s machine learning is the result of the two stages running in parallel, rather than excluding articles on the basis of their predicted relevancy score alone.
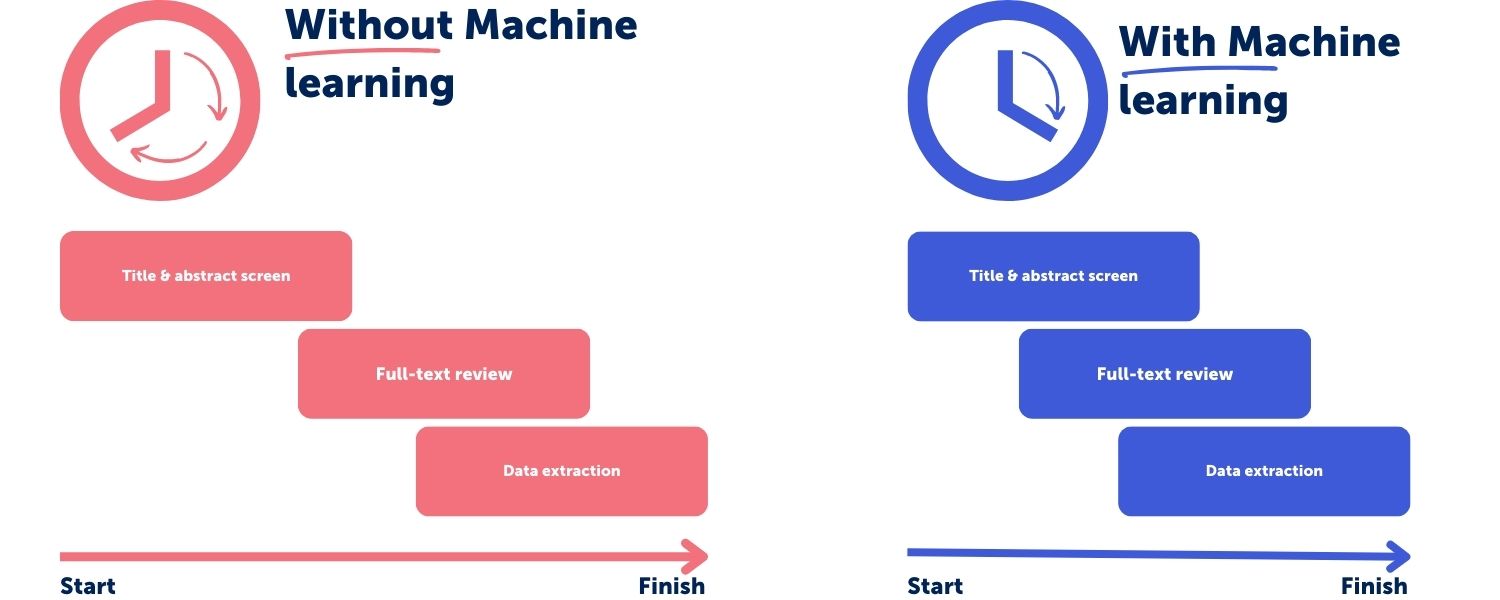
Rigorous testing for peace of mind.
Before implementing the machine learning algorithm, it was important to us to test it thoroughly to ensure it was working correctly and providing accurate results. This rigorous testing was done to give users peace of mind that the algorithm is reliable and will provide the desired results. With the right testing and implementation, machine learning can be a powerful tool for improving the efficiency and accuracy of study screening and at Covidence we are confident this has been achieved.
This new feature will certainly save you a lot of time with the ability to complete tasks faster and more efficiently. At Covidence we are always striving to develop features that will improve your workflow and bring more trustworthy research into the world, and active learning is a game changer for doing just that.
Well, what are you waiting for? Try it for yourself!
Release Notes: December 2022 – Title and Abstract Screening Using Machine Learning